
- This event has passed.
Advancements in Bayesian Neural Networks to Enhance Predictive Modeling for Civil Engineering Applications
February 22 @ 11:00 - 14:00
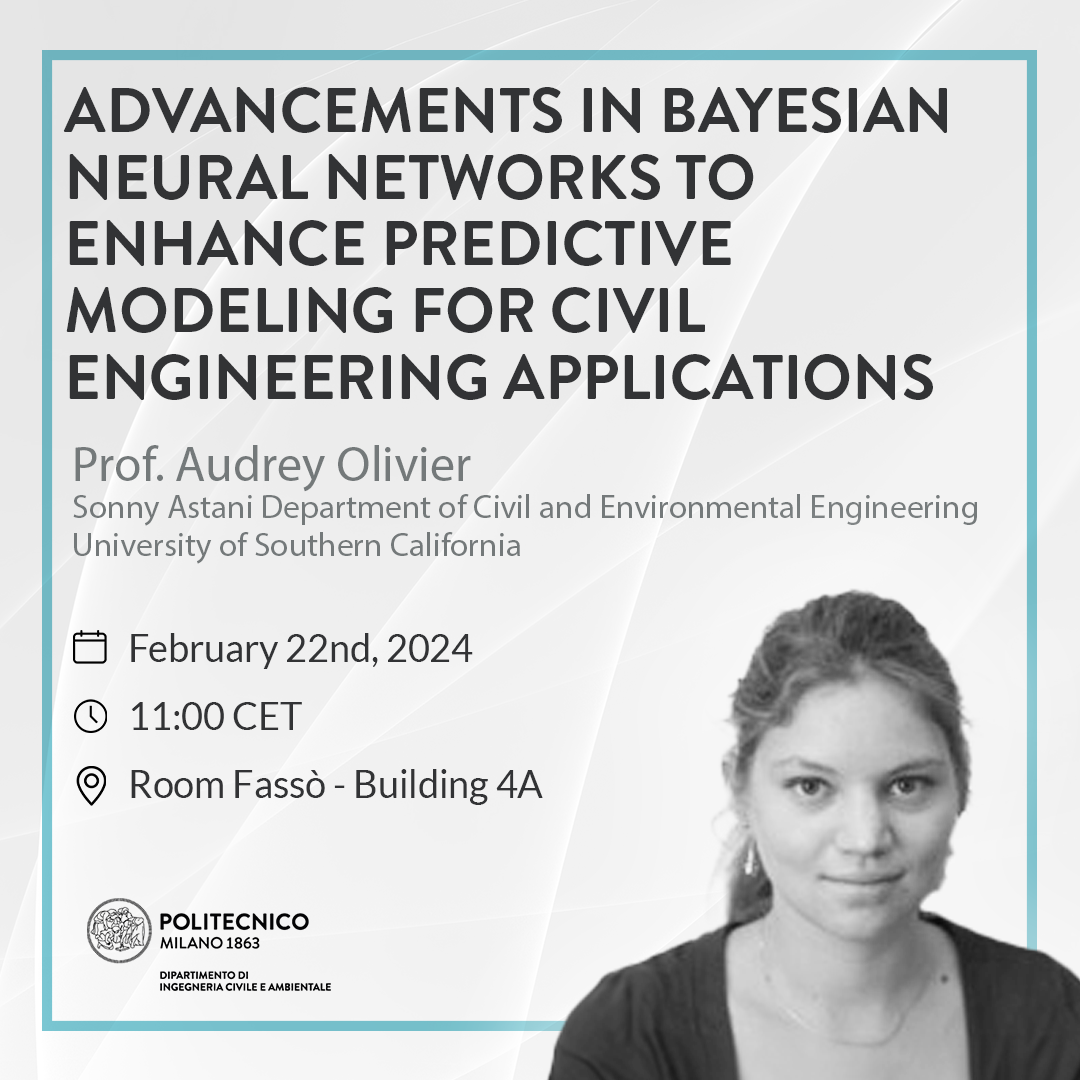
On February 22nd, at 11:00 AM, a seminar titled “Advancements in Bayesian Neural Networks to Enhance Predictive Modeling for Civil Engineering Applications“ will take place in Room Fassò (Building 4A – Piazza Leonardo da Vinci, 32 – Milan).
The seminar will be conducted by Prof. Audrey Olivier (Sonny Astani Department of Civil and Environmental Engineering, University of Southern California).
Abstract
The conjuncton of data mining and physics-based modeling holds great potential to help design, monitor and optimize civil infrastructure systems. Machine learning (ML) tools can be used for a variety of civil engineering applications, from monitoring the health of structures using sensing data to building efficient surrogates to accelerate mechanistic modeling. The use of ML models for scientific and engineering applications presents unique challenges. Engineering datasets are often noisy, sparse and imbalanced, due to the inherent randomness of the underlying physical processes and constraints on data collection. ML predictors must also assimilate physics-based knowledge and intuitions to improve accuracy and generalization away from training data. Finally, ML models must embed robust prediction of uncertainties to improve trustworthiness for high-consequence decision-making. Framing ML training within a Bayesian inference framework allows for a robust quantification of both aleatory and epistemic uncertainties that arise from data inadequacies, integration of physics intuitions through prior design, and assessment of the model’s confidence in its predictions. However, due to the high-dimensionality and non-physicality of parameters that characterize typical ML models such as neural networks, application of Bayesian methods in this context raises several challenges, from prior and likelihood design to posterior inference. In this talk I will present some of the methods we have developed for approximate Bayesian learning of neural networks that integrate meaningful physics-driven priors. I will illustrate the benefits of these methods through a variety of example applications in civil engineering, from surrogate training to accelerate materials and structural modeling, contingency analysis in power grid systems, or ambulance travel time prediction in a dense urban network to help optimize emergency medical services.
Short Bio
Dr. Olivier holds a Diplôme d’Ingénieur from École Centrale de Nantes, France, and a Ph.D. in Civil Engineering and Engineering Mechanics from Columbia University, USA. She held a postdoctoral appointment at Johns Hopkins University before joining the Sonny Astani Department of Civil and Environmental Engineering at the University of Southern California as an Assistant Professor in Fall 2021. Dr. Olivier’s research aims to predict and monitor civil infrastructure systems behavior under uncertainty, by combining innovations in probabilistic data analytics and mechanistic modeling. Applications span various scales, from systems to structures to materials, and include development of adaptive Bayesian filters for identification of dynamical structural systems, probabilistic surrogate models to accelerate multi-scale materials simulations or Bayesian graph neural networks for contingency analysis of power grids. Additional information available at: https://www.audreyolivier.com/; https://viterbi.usc.edu/directory/faculty/Olivier/Audrey .